The NIH and NSF
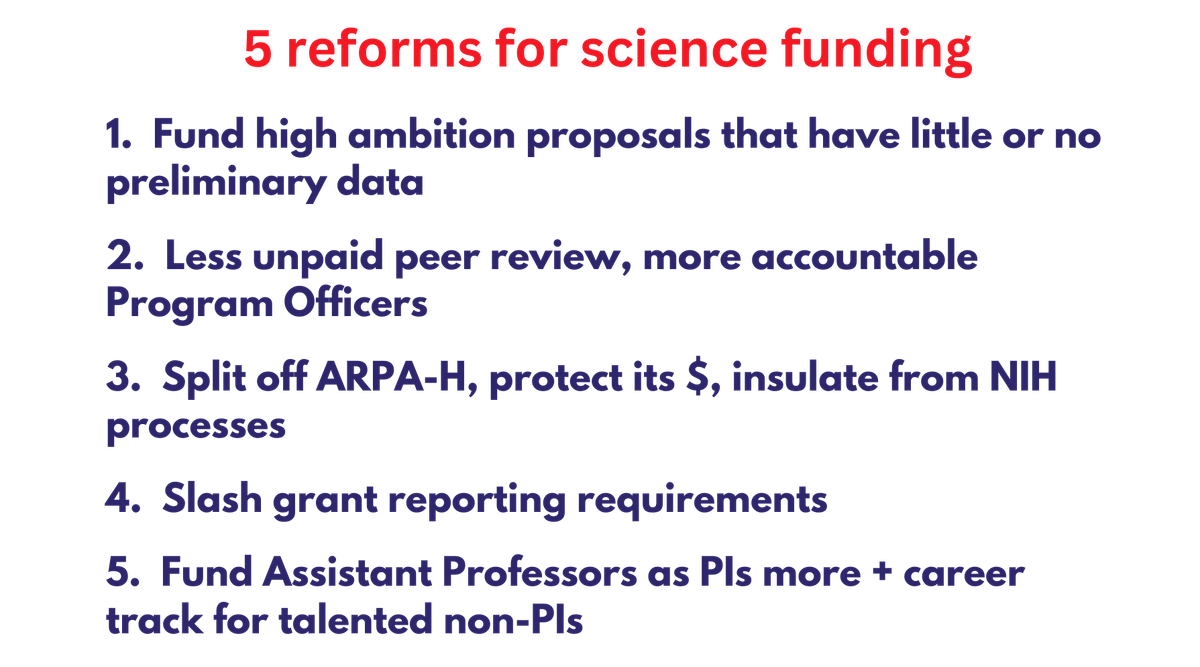
This decade brought two inventions that will prevent a million deaths in America. One came from a German company who partnered with Pfizer to make Comirnaty available nine months into the COVID pandemic, becoming the most used vaccine in the U.S. at its peak (vs Moderna’s and J&J’s). The other came from a Danish company: Ozempic/Wegovy reduces the risk of heart attacks and strokes for people with diabetes, and helps obese people lose weight.
America funds public research largely via two federal agencies: the NIH and the NSF. Together, those agencies cost us just 1/10th the Defense budget, or 1/20th of social security spending. Yet if we end up curing cancer before the Germans, or making quantum computers before the Danes, the seeds will likely come from NIH and NSF projects. In this post, I add my two cents to the discussion of how to improve the two agencies, and where it’s time to start fresh without them.
My perspective comes from working in science philanthropy, which lives partly in reaction to gaps left open by the NIH and NSF. Many of the scientists we support at Open Philanthropy are employed at American universities: their careers are mostly funded by those universities, and by the NIH. We intentionally try only to fund projects the NIH and NSF haven’t, or can’t, or wouldn’t – meaning I have a poacher’s respect for their lush forests and a poacher’s obsession with their blindspots. Some of my own blindspots in this post come from being an outsider to both institutions: I have not had to navigate the tradeoffs they face from the inside. It is always easier to criticise, and I hope my missteps will be forgiven as lack of knowledge, not lack of empathy.
[This post is personal, and does not represent the views of my employer. I have never received an NIH/NSF grant. That said, I learned in my twenties that my grandfather was the Executive Secretary of the National Science Board, and assistant to the first NSF Director, Alan Waterman, when the NSF was founded in 1950 – so any bias towards NSF is borne from blood:]
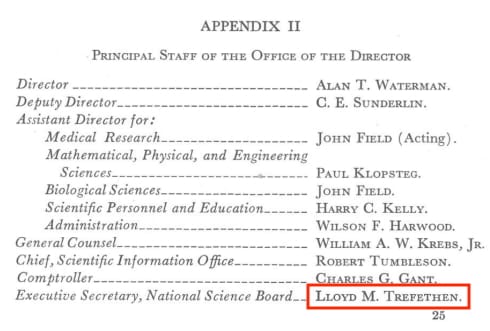
Tradeoffs in research funding, if you hold total $ fixed
There should clearly be much more money flowing through the research system, in my view, than there is today. The mercenary financial reason is science boosts long run growth of the economy, and thereby tax revenues, so pays for itself with surplus to spare. The human reason is that civilian research is only 1% of the federal budget and we haven’t cured Alzheimer’s or depression yet. 1% is the same amount as America spends on dialysis. Dialysis alone.
(Is there an ARPA-H Program Manager working on moonshots to replace/reduce the need for dialysis yet? OK, I just googled after typing that sentence, and there is; ARPA-H, you beat me again. But why not go one step further than improving kidney donation success rates? Creating organs for transplant from induced pluripotent stem cells would be an extremely difficult technical problem – but perhaps achievable, and what a life goal to take on!)
Some research with risks of harm should be stopped.[3] But most research has more risk of benefiting people than harming them. And more money could fund more research. 85% of NIH project grant applications on aging will get rejected this year, and 90% on infectious disease will, due to budget limitations. That rejection is for the scientists who stuck around and kept applying. In our current system, many talented people who wanted to work in science and engineering already left to work at investment banks.
That all sounds like an easy pitch to me to increase funding for public research, but clearly I must be missing something, so allow me some pessimism for this post. Hold the money fixed. How can we improve the system through which it flows?
One lens to approach that question is to put decision-making tradeoffs on axes, and debate which direction we should head from here:
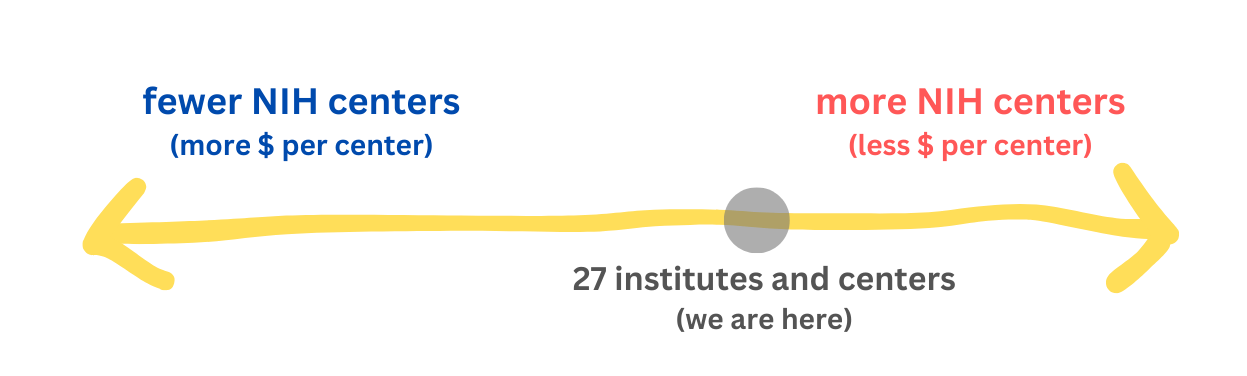
Often, it is hard to know which direction would be better, and the quality of evidence that would bear on the question is low. But sometimes, there is a fair amount of consensus among people who study scientific progress. Luckily for me, in addition to traditional fields of philosophy and sociology of science, science and technology studies, and the economics of innovation, there is a new wave of energy around metascience, attempting to answer questions like this head on. (Indeed our Innovation Policy program, run by my colleague Matt Clancy, funds metascience research to try and help.) I have learned much from this community, and hope I don’t misreport their views below. This post covers the easier cases for reform, where considerable evidence has been collected and debated, and it's time to act.
High consensus reforms
1. Higher risk ambitious science ⬆️ vs incremental science that is likely to work ⬇️
- Evidence and argument: Risk-taking harms your grant renewal chances. Study panels are risk averse about new ideas: “work that builds on brand new ideas is funded at a lower rate than is work that builds on ideas that have had a chance to mature at least 5-10 years” (coauthor = Jay Bhattacharya). The costs of risk aversion are a focus of Donald Braben’s book; summary slides of the book here. Lit reviews show biases against risky research and conservatism in science. This overview of research conservatism by Matt Faherty for New Science discusses what factors drive it at the NIH.
- In 2017, we ran a “second chance” program at Open Philanthropy (before my time; I joined in 2018) for applicants to NIH’s Transformative Research Award (TRA) program – a category designed for ambitious projects that are too risky to attract conventional NIH project (“R01”) support. There was good stuff in there that the NIH had rejected due to limited funds, and we funded 4 grants. 4 may not sound like many, but the NIH only funds 6-10 transformative research grants each year!
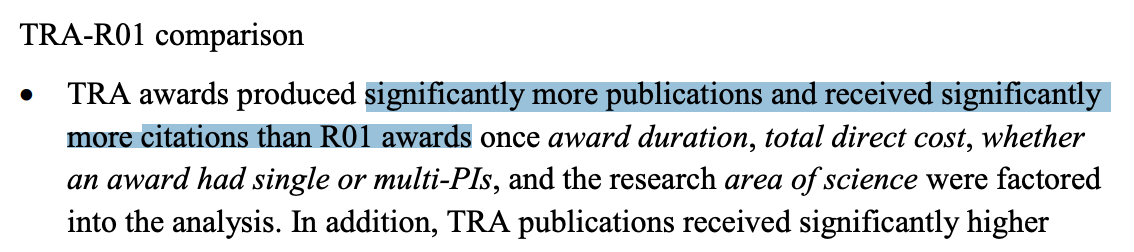
- Ideas for fixes: (a) Across all grant categories, require less preliminary data to give grant applications a strong score, and tell study section reviewers they can give low scores to unambitious proposals even if the proposals have great preliminary data. (NIH's TRA already has no requirement for preliminary data; we can pilot beyond.) (b) NIH evaluate “Rigor and Feasibility” for sufficiency (as with “Expertise and Resources”) instead of on a 1-9 scale, and aggregate only the “Importance of the Research (Significance, Innovation)" numerical scores across reviewers. This will, roughly speaking, bring NIH closer to NSF's stated evaluation criteria, where feasibility/expertise/resources are secondary rather than primary features of the assessment. (c) Fund more winners in the transformative research grant category each year, vs regular R01s. (d) Expand “people” grants like MIRA that allow more flexibility for scientists, vs regular R01s. (e) NIH copy NSF’s experiment with golden tickets for peer reviewers, to bump up high variance grants. (f) NIH directors encourage levels of discretion for POs closer to NSF’s, so staff POs can more frequently bump proposals with lower average scores over the funding cutoff line (“payline”), especially if the reviewer scores are high variance; assess performance of those grants as part of PO promotion packages. And, relatedly…
2. Program Officer/Program Director power ⬆️ vs peer review panel power ⬇️
- Similar to: decisions by directly-responsible individuals ⬆️ vs decisions by shared-responsibility committees ⬇️
- See lit review on taste vs peer review. Plus, evidence like: “ARPA-E program directors prefer to fund proposals with greater disagreement among experts, especially if at least one reviewer thinks highly of the proposal. This preference leads ARPA-E to fund more uncertain and creative research ideas”.
- This is one where the NSF and NIH differ a bit: my understanding from the outside is that Program Officers at NSF – and especially Program Directors of particular divisions – have more discretion to boost proposals they like that didn’t get the highest average score. NIH POs tend to stick to the scores: if a proposal gets a high enough average score from peers, it gets funded; if not, you’re almost always out of luck (unless you're a senior scientist with a great relationship with your PO).
- At the other end of the extreme, DARPA, ARPA-H, and ARPA-E Program Managers (PMs) have significant discretion to fund grantees (“performers”) based on what they believe will move the field/problem they’re focused on forward the most. This can lead to "petty tyrant" problems if the wrong PM represents a significant share of funding in a field for too long and micromanages grantees, but in the best case gets us things like the internet.
- Scientists care about objectivity in their work, and the funding system is designed partly to reflect this – publications get peer reviewed to assess their robustness and contribution to the field, so grants should too, right? In my view this has its place but has gone too far. Science should try to be retroactively objective, not prospectively objective. It is easier to read results off a chart once the data exists, or to replicate an experiment in your own lab once you can read the protocol that worked somewhere else, than to figure it all out the first time. Plus, there are well known issues with peer reviewing grants that don’t apply as much to PO-/PM-led grantmaking – peer review is not unbiased: (a) you’re getting assessed by your scientific “competitors”, who often have their own axe to grind; (b) consensus and average scores leads to risk aversion (see above), and (c) the more decision-makers there are, the slower the decision is – you have to convene a panel, and everyone’s busy. Last year we had to wait a month for a co-funder of a project to find a third peer reviewer for the grant proposal we were assessing together – despite everyone knowing in their hearts it was a great proposal (which, sure enough, we both funded).
- Ideas for fixes: (a) Require 2 external reviewers rather than 3 in more cases. (b) Encourage NIH POs to deviate from paylines more often. (c) Possibly make it easier for POs to reject and accept applications without review, to allocate review time better (I believe this is sort of possible at NSF if you introduce a “pre-application” stage?). (d) Have more funding go through Program Manager-driven systems than peer review systems, generally, relative to the 2024 default (this can go too far, we’re just not near "too far" yet). Which brings me to…
3. Share of funding via NIH Institutes ⬇️ vs other science funding institutions ⬆️
- One thing almost everyone in the metascience community agrees on is that there should be more variety in the mechanisms, available career tracks, and cultures pursuing the scientific enterprise. This point is most widely explored in Michael Nielsen and Kanjun Qiu’s 2022 essay A Vision of Metascience. Homogeneity/concentration in any system makes it difficult for best practices to spread.
- (That said, even the most agreed upon reforms have dissenters! Here is an interesting thought experiment from Jose Ricon that it’s not so simple.)
- So, create a new government science funder, with different funding methods? Sounds great to me. The good news is… we just did: ARPA-H. However, ARPA-H is currently attached to the Borg, and last I heard the House is proposing to merge it with two other arms of the NIH and cut its budget.
- Idea for a fix: Congress should spin off ARPA-H from the NIH + protect/grow its funding over time.
4. More reporting/paperwork ⬇️ vs less reporting/paperwork ⬆️
- OK, who is asking for more paperwork? Isn't this one a false dichotomy? Well, what politicians and voters (e.g. me) want, that gets turned into more paperwork, is accountability. We want accountability because it's public money, and we live in a democracy. But accountability is simply not achieved with the current onerous reporting system – what is achieved is high administrative expenditures and slow science. As one NIH-funded scientist friend put it: “The reporting has minimal accountability because unless you are wildly unproductive or drastically mismanage your grant funds (or don't report) no one says anything.”
- Slashing reporting requirements would save scientists time, and save the NIH money in indirect payments on grants – admin support at universities is partly for grant administration required by funders.
- Here’s a real headline from April this year, about UC San Diego: "One Scientist Neglected His Grant Reports. Now U.S. Agencies Are Withholding Grants for an Entire University". The scientist in question had retired, and hadn’t submitted final technical reports for two NIH awards. UCSD has >1,000 ongoing NIH grants, totaling over $600 million.
- Ideas for fixes: I don’t know the systems well enough to get specific on this one, so will leave it to others like Stuart Buck. That said, I suspect the requirements have to be slashed all over, especially when it comes to backend reporting that rarely has surprises in it and leads to high administration costs on both universities and the agencies (someone’s got to read all that paperwork). In 1954, the first NSF secretary left the NSF in part because the paperwork requirements were getting too burdensome, drifting from its original 1950 mission…
5. Early career Principal Investigators get their own grants ⬆️ vs scientists live off of other people’s grants until age 35+ ⬇️
- ("Principal Investigator" = PI = lead scientist)
- Evidence and argument: average age of first time investigators keeps rising, share to >66yos vs <35yos has inverted over the last few decades. Striking graph. Alexey Guzey’s writings. Lit review on age and the impact of innovations.
- The NIH and NSF are both aware of and working on this problem, but their efforts so far haven’t seemed to make much of a dent. The change has to be drastic here, to avoid hemorrhaging more talent to unrelated industries over public science.
- Ideas for fixes: (a) NIH and NSF automatically match university starting packages for Assistant Professors. Hiring a professor is a huge decision that university departments already put lots of effort into, and this would help reward that effort in a decentralised way (i.e. leaning on many institutions across America and their view of what’s needed, not Bethesda's). (b) Again, require less preliminary data – that requirement is a clear bias towards people who’ve run labs for a long time! (c) Probably expand further the K99/R00 category at NIH and CAREER grants at NSF. (d) Experiment with more blinded grant applications for R01s, where the scientist’s name isn’t attached (the transformative research award does this already) – often, younger PIs with less experience are considered the risky part of a proposal by the panel. (d) Probably raise postdoc salaries and/or offer additional flexible fellowship funding (doesn’t help with taking on students, but does help with flexibility for your own research). (e) Probably encourage postdocs to apply for grants more themselves or as co-PIs; this happens in the UK and many European countries, though the idea frequently doesn’t compute in the current American system. (f) Pilot more grant pools where only early career scientists can apply, since part of the skew of who gets money comes from differences in who applies, rather than which applicants get selected.
Thank you to the many insiders and outsiders to these agencies I’ve spoken to over the years, especially to academics who still have enough hope to complain. Thank you in particular to Heather Youngs, Chris Somerville, Katharine Collins, Matt Clancy, Jordan Dworkin, Melanie Basnak, Brandon Wilder, Caleb Watney, Anne Trefethen, Nick Trefethen, Michael Nielsen, Seemay Chou, and Prachee Avasthi. I expect none of them agrees with everything I’ve written. Any good ideas in here are likely theirs; any errors are my own.
[1] Preventing 1 million deaths sounds like a lot, even for miracle drugs – where did I get those numbers? Starting with Comirnaty: it is very difficult to model a complicated, nonlinear pandemic against reasonable counterfactuals, but one attempt estimates that COVID vaccines prevented 18 million hospitalisations and 3.2 million deaths in America in their first two years of use. The BioNTech/Pfizer vaccine was the most used vaccine in the U.S. during that period (over 1/3 of doses), so I think it is fair to attribute 1 million deaths averted to it. The case of Ozempic/Wegovy is a little trickier, and I'm more at risk of exaggerating. GLP-1 agonists have not been available for long, so it's hard to predict how much use they'll get long term – and there are plenty of competitor drugs from other companies now (including American companies), so not all deaths averted by the drug class will be attributable to Ozempic/Wegovy. Then people taking GLP-1s today are less likely to die over the coming decades, so it will take longer to measure the results too. I have not come across clear estimates for expected lives saved from the drugs. This paper suggests they could prevent ~40k deaths per year in the US, which would (crudely/linearly) mean 1 million with 25 years of use. This preprint suggests ~900k deaths per year globally. Part of the reason I felt comfortable sticking by the "1 million in America" claim is that, so far, clinical researchers seem to be finding further mortality-reducing benefits from GLP-1s on other diseases beyond diabetes and obesity, and beyond cardiac events – not yet measured in the modeling. So: in a decade's time, the opening of this blog post may turn out to have been false and too rosy, or not rosy enough, with respect to the impact of GLP-1s in America.
[2] Are there other inventions from the last 10 years that will prevent 1 million American deaths? I haven’t attempted to run the numbers, but the next tier that come to mind are: (iii) PCSK-9 inhibitors: Merck’s oral daily that was voted 2023 Molecule of the Year looks to be as good as injectable PCSK-9s and as statins at reducing heart disease risk, without as many side effects; (iv) the new RSV vaccines and monoclonals, which have been brought to market by multiple companies in the last few years after a structural biology breakthrough from an NIH intramural lab in 2013; and (v) Zoom + Google Meet, which allowed many white collar employees to work from home during the worst of the COVID pandemic. To our credit, these were all (mostly) invented in America. Plus, much of the research that enabled Comirnaty and Ozempic to reach the finish line was completed by U.S. researchers – Denmark's got our back, and we've got theirs. 🇩🇰
[3] Which types of research should be treated very carefully, or stopped, for risk of harm? Speaking just for myself I’d say: gain-of-function research, if the “function” is increased transmissibility or pathogenicity of whole viruses. Nucleic acid synthesis machines/providers should require checks against a database of known pathogens, and logging of all requested sequences in case new harmful proteins not seen in nature are designed with the help of AI. If you’re thinking of running experiments of these 7 types, think carefully whether it’s worth it – some aren’t allowed and will get blocked by your IRB; for the ones that are allowed, remember that Russia is reopening old labs previously used for bioweapons research, and they can read any public research too.